Food delivery is a unique high-stakes quick commerce service, particularly because plenty of factors influence successful delivery, and there's barely any time to rectify any mishaps in this process. This is why at Jumia, we’ve emphasized data analytics as a solid approach to anticipating any eventualities and preventing them or having contingencies in place.
However, this effort isn't straightforward since we have to harmonize the customer's needs with those of riders and the organization's needs (specifically the business end). Accordingly, let’s explore the major desires of each side and show how data analytics addresses them:
Customers – Primarily, customers want a speedy service that's also affordable. They want to rest assured that regardless of their location in the area covered by Jumia Food, they can have their meal on time without spending too much on delivery fees.
Delivery Riders – As always, our riders want to ensure they can deliver as many orders as possible within a single shift to maximize their earnings.
Jumia – Regarding quick commerce, the margins on food delivery remain slim, so while profit is greatly appreciated, the primary focus is growth. Numerous sizable markets across Africa are still untapped, and we trust that the customer base for our food delivery service can continue growing rapidly while we improve quality and moderate customer acquisition costs.
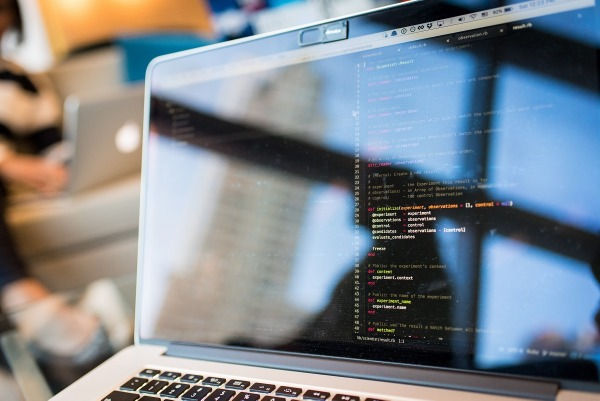
Using data science to improve food delivery comprehensively
We've started several data science projects to create a better experience for the stakeholders above, and these include:
Accurate Estimated Time of Arrival (ETA)
When customers browse through different restaurants and other vendors, they should know how long it will take for their food to arrive. While the simpler way is to have generic estimates based on the distance between the vendor and the customer, the reality may be quite different from time to time, leaving customers frustrated when there are delays.
This is why we’ve gone a step further by analyzing the time it takes the vendor to prepare that order, previous delivery times associated with that vendor when covering a similar distance to the customer, and many other factors. Subsequently, we can offer a more accurate ETA for each vendor, which makes customers more confident and more likely to order from various vendors since they know the food will arrive within the stated time.
Strategic Order Allocation
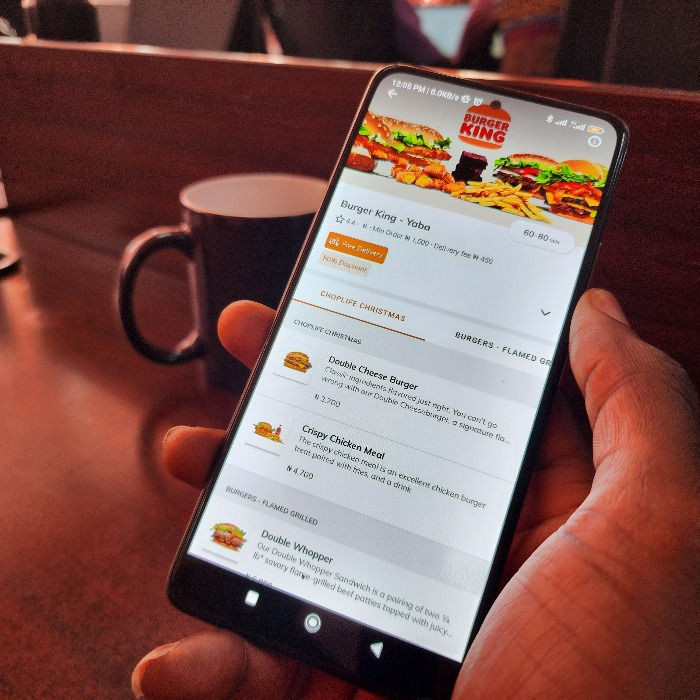
One of the greatest puzzles in food delivery is deciding which rider should pick up an order once it’s ready. Remember, we don't have much time to choose since most meals are prepared quickly. More importantly, the rider nearest to the vendor when the order is almost ready isn't always the best choice since they might be delivering to someone in another location.
In fact, another rider who's slightly further from the vendor but is just about to finish dropping off an order might be their better choice. In that sense, we use data analytics to go beyond basic metrics like a rider’s proximity to the vendor. We consider their current delivery status and which other pending orders might be a better fit. This enables us to ensure that no rider arrives at the vendor's location too early or too late, allowing them to maximize orders delivered in one shift.
Forecasting Riders Needed
At the end of each day, we can use software to determine the number of orders delivered within a specific area. And thanks to cutting-edge data analytics tools, we can predict the order volumes for different areas in shorter timeframes.
By knowing the number of orders we will handle in a certain area in the next day or even the next couple of hours, we can quickly determine the number of riders we will need in a specific area at any given time. This is crucial since each rider can work a limited amount of time before their performance drops.
Therefore, comparing order volume data with rider operational hours enables us always to have the right number of riders in every area so that every order is delivered on time. It also helps us deploy and redirect riders to areas where they’ll maximize orders delivered per shift. Remember that data analytics tools help us track all these changes every second to make real-time decisions.

Evaluating Rider Performance and Refining Recommendations
When riders pick up or deliver food, they can face challenges beyond their control that cause delays and diminish the customer’s experience. These include weather changes, traffic jams, diversions due to road works, unfavorable terrain, insufficient addressing systems/street labeling to complement GPS, and much more.
Accordingly, we can use data analytics to detect patterns in orders associated with specific areas and then combine that with rider and customer feedback for a fairer assessment of a rider's performance. This also helps us refine recommendations to suggest the vendor option where we know we will offer the best delivery experience possible.
Ultimately, we aim to provide a consistent experience regardless of the vendor selected or the customer’s location within our covered area. To achieve this, we must have all pertinent data points regarding factors influencing order delivery. Furthermore, they should be accurate and available on time in an environment where we can compare them and derive actionable insight.
Lastly, for factors beyond our control, we need to foresee these changes based on previous performance and current events, which also requires a robust analytics stack. Fortunately, we trust our data specialists to keep working on projects that drive solid decision-making and satisfactory experiences for customers, riders and other stakeholders.
**************************
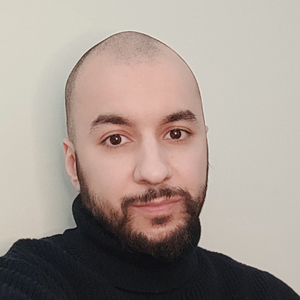
Ivo Nogueira
Lead Data Scientist, Jumia Porto Tech Center
Ivo is the Lead Data Scientist for On-Demand Services (ODS). He has worked in multiple fields, having acquired expertise in E-commerce and Retail through his years working at Jumia, Glovo, Farfetch, and Sonae.
Ivo holds two master's degrees in Economics and Data Analytics, and he is currently working on a Ph.D. in Applied Mathematics. He is also a University Professor of Data Science at ISAG - European Business School..
Commentaires